이 백서는 Colbert Architecture를 기반으로하고 Modernbert Foundation을 통합하는 새로운 모델 인 GTE-Moderncolbert-V1을 소개합니다.
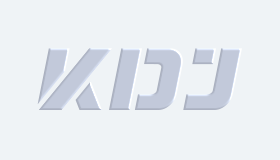
Researchers from LightOn AI have presented GTE-ModernColBERT-v1, a model that builds upon the ColBERT architecture and integrates the ModernBERT foundation developed by Alibaba-NLP. Their aim was to distill knowledge from a base model and optimize it on the MS MARCO dataset, hoping to overcome limitations related to context length and semantic preservation. The model was trained using 300-token document inputs but demonstrated the ability to handle inputs as large as 8192 tokens, making it suitable for indexing and retrieving longer documents with minimal information loss. This work was deployed through PyLate, a library that simplifies the indexing and querying of documents using dense vector models. The model performs token-level semantic matching using the MaxSim operator, which evaluates similarity between individual token embeddings rather than compressing them into a single vector.
Lighton AI의 연구원들은 Colbert 아키텍처를 기반으로하고 Alibaba-NLP가 개발 한 ModernBert Foundation을 통합하는 모델 인 GTE-Moderncolbert-V1을 발표했습니다. 그들의 목표는 기본 모델에서 지식을 증류하고 MS Marco 데이터 세트에서 최적화하여 상황 길이 및 시맨틱 보존과 관련된 한계를 극복하기를 희망하는 것이 었습니다. 이 모델은 300 번의 문서 입력을 사용하여 교육을 받았지만 8192 개의 토큰의 입력을 처리 할 수있는 기능을 보여 주었으므로 최소한의 정보 손실로 더 긴 문서를 인덱싱하고 검색하는 데 적합합니다. 이 작업은 조밀 한 벡터 모델을 사용하여 문서의 인덱싱 및 쿼리를 단순화하는 라이브러리 인 Pylate를 통해 배포되었습니다. 이 모델은 MaxSim 연산자를 사용하여 토큰 수준의 시맨틱 매칭을 수행하며, 이는 단일 벡터로 압축하는 대신 개별 토큰 임베딩 사이의 유사성을 평가합니다.
GTE-ModernColBERT-v1 transforms text into 128-dimensional dense vectors and uses the MaxSim function for computing semantic similarity between query and document tokens, preserving granular context and enabling more refined retrieval. It integrates with PyLate’s Voyager indexing system, which manages large-scale embeddings using an efficient HNSW (Hierarchical Navigable Small World) index. Once documents are embedded and stored, users can retrieve the top-k most relevant documents using the ColBERT retriever. This process supports full pipeline indexing and lightweight reranking for first-stage retrieval systems. PyLate offers flexibility in modifying document length during inference, allowing users to handle texts much longer than the model was originally trained on, an advantage rarely seen in standard embedding models.
GTE-MODERNCOLBERT-V1은 텍스트를 128 차원 밀도 벡터로 변환하고 쿼리와 문서 토큰 간의 의미 론적 유사성을 계산하기 위해 최대 값 기능을 사용하여 입상 컨텍스트를 유지하고보다 세련된 검색을 가능하게합니다. Pylate의 Voyager 인덱싱 시스템과 통합되어 효율적인 HNSW (계층 적 항해 가능한 작은 세계) 인덱스를 사용하여 대규모 임베드를 관리합니다. 문서가 포함되고 저장되면 사용자는 Colbert Retriever를 사용하여 가장 관련성이 높은 문서를 검색 할 수 있습니다. 이 프로세스는 1 단계 검색 시스템에 대한 전체 파이프 라인 인덱싱 및 가벼운 재평가를 지원합니다. Pylate는 추론 중에 문서 길이를 수정하는 데 유연성을 제공하므로 사용자는 원래 모델보다 훨씬 더 긴 텍스트를 처리 할 수 있습니다. 표준 임베딩 모델에서는 거의 보이지 않습니다.
On the NanoClimate dataset, the model achieved a MaxSim Accuracy@1 of 0.360, Accuracy@5 of 0.780, and Accuracy@10 of 0.860. Precision and recall scores were consistent, with MaxSim Recall@3 reaching 0.289 and Precision@3 at 0.233. These scores highlight the model’s capability to retrieve accurate results even in longer-context retrieval scenarios.
Nanoclimate 데이터 세트 에서이 모델은 0.360의 1의 최대 정확도, 0.780의 정확도 및 0.860의 정확도를 달성했습니다. Maxsim Recall@3은 0.289에 도달하고 3 번의 정밀도는 0.233에서 정밀 및 리콜 점수가 일관되었습니다. 이 점수는 텍스트 장기 검색 시나리오에서도 정확한 결과를 검색 할 수있는 모델의 기능을 강조합니다.
When evaluated on the BEIR benchmark, GTE-ModernColBERT outperformed previous models, including ColBERT-small. It scored 54.89 on the FiQA2018 dataset, 48.51 on NFCorpus, and 83.59 on the TREC-COVID task. The average performance across these tasks was significantly higher than baseline ColBERT variants. Notably, in the LongEmbed benchmark, the model scored 88.39 in Mean score and 78.82 in LEMB Narrative QA Retrieval, surpassing other leading models such as voyage-multilingual-2 (79.17) and bge-m3 (58.73). These results suggest that the model offers robust generalization and effective handling of long-context documents, outperforming many contemporaries by almost 10 points on long-context tasks. It is also highly adaptable to different retrieval pipelines, supporting both indexing and reranking implementations, making it a versatile solution for scalable semantic search.
BEIR 벤치 마크에서 평가되면 GTE-Moderncolbert는 Colbert-Small을 포함한 이전 모델을 능가했습니다. FIQA2018 데이터 세트에서 54.89 점, NFCORPUS에서 48.51, TREC-Covid 작업에서 83.59 점을 얻었습니다. 이러한 작업의 평균 성능은 기준선 Colbert 변형보다 상당히 높았습니다. 특히, Longembed 벤치 마크에서, 모델은 평균 점수 88.39 점, LEMB 내러티브 QA 검색에서 78.82 점을 얻었으며 항해-다중 언어 2 (79.17) 및 BGE-M3 (58.73)과 같은 다른 주요 모델을 능가했습니다. 이 결과는이 모델이 장기 텍스트 문서의 강력한 일반화 및 효과적인 처리를 제공하여 장기 텍스트 작업에서 많은 동시대 인을 거의 10 점으로 능가한다는 것을 시사합니다. 또한 인덱싱 및 재고 구현을 모두 지원하여 확장 가능한 시맨틱 검색을위한 다목적 솔루션이됩니다.
Several Key Highlights from the Research on GTE-ModernColBERT-v1 include:This research provides a meaningful contribution to long-document semantic retrieval. By combining the strengths of token-level matching with scalable architecture, GTE-ModernColBERT-v1 addresses several bottlenecks that current models face. It introduces a reliable method for processing and retrieving semantically rich information from extended contexts, significantly improving precision and recall in longer-context retrieval scenarios.
GTE-Moderncolbert-V1에 대한 연구의 몇 가지 주요 하이라이트는 다음과 같습니다.이 연구는 장기 문서 시맨틱 검색에 의미있는 기여를 제공합니다. GTE-Moderncolbert-V1은 토큰 수준의 강점을 확장 가능한 아키텍처와 결합하여 현재 모델이 직면하는 몇 가지 병목 현상을 해결합니다. 확장 된 컨텍스트에서 의미 적으로 풍부한 정보를 처리하고 검색하는 신뢰할 수있는 방법을 소개하여 장기 텍스트 검색 시나리오에서 정밀도를 크게 향상시키고 리콜을 크게 향상시킵니다.