このペーパーでは、Colbert Architectureに基づいてModernbert Foundationを統合する新しいモデルであるGTE-ModernColbert-V1を紹介します。
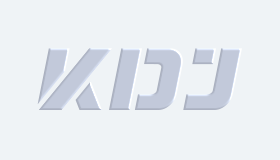
Researchers from LightOn AI have presented GTE-ModernColBERT-v1, a model that builds upon the ColBERT architecture and integrates the ModernBERT foundation developed by Alibaba-NLP. Their aim was to distill knowledge from a base model and optimize it on the MS MARCO dataset, hoping to overcome limitations related to context length and semantic preservation. The model was trained using 300-token document inputs but demonstrated the ability to handle inputs as large as 8192 tokens, making it suitable for indexing and retrieving longer documents with minimal information loss. This work was deployed through PyLate, a library that simplifies the indexing and querying of documents using dense vector models. The model performs token-level semantic matching using the MaxSim operator, which evaluates similarity between individual token embeddings rather than compressing them into a single vector.
Lighton AIの研究者は、Colbert Architectureに基づいて構築され、Alibaba-NLPが開発したModernbert Foundationを統合するモデルであるGTE-ModernColbert-V1を発表しました。彼らの目的は、知識を基本モデルから蒸留し、MS Marco Datasetで最適化し、コンテキストの長さと意味保存に関連する制限を克服することを望んでいました。このモデルは、300トークンのドキュメント入力を使用してトレーニングされましたが、8192トークンの入力を処理する機能を実証し、情報損失を最小限に抑えてより長いドキュメントのインデックス作成と取得に適しています。この作業は、密なベクトルモデルを使用してドキュメントのインデックス作成とクエリを簡素化するライブラリであるPylateを介して展開されました。このモデルは、MaxSimオペレーターを使用してトークンレベルのセマンティックマッチングを実行します。これは、単一のベクトルに圧縮するのではなく、個々のトークン埋め込みの類似性を評価します。
GTE-ModernColBERT-v1 transforms text into 128-dimensional dense vectors and uses the MaxSim function for computing semantic similarity between query and document tokens, preserving granular context and enabling more refined retrieval. It integrates with PyLate’s Voyager indexing system, which manages large-scale embeddings using an efficient HNSW (Hierarchical Navigable Small World) index. Once documents are embedded and stored, users can retrieve the top-k most relevant documents using the ColBERT retriever. This process supports full pipeline indexing and lightweight reranking for first-stage retrieval systems. PyLate offers flexibility in modifying document length during inference, allowing users to handle texts much longer than the model was originally trained on, an advantage rarely seen in standard embedding models.
GTE-ModernColbert-V1は、テキストを128次元密度のベクトルに変換し、クエリとドキュメントトークンの間のセマンティックな類似性を計算するためにMaxSim関数を使用し、粒状コンテキストを保存し、より洗練された検索を可能にします。 PylateのVoyager Indexing Systemと統合されており、効率的なHNSW(階層ナビゲーションスモールワールド)インデックスを使用して大規模な埋め込みを管理しています。ドキュメントが埋め込まれて保存されると、ユーザーはColbert Retrieverを使用してTop-Kのほとんどの関連するドキュメントを取得できます。このプロセスは、第1段階の検索システムの完全なパイプラインインデックスと軽量の再ランキングをサポートします。 Pylateは、推論中にドキュメントの長さを変更する柔軟性を提供し、ユーザーがモデルよりもはるかに長くテキストを処理できるようにします。これは、標準の埋め込みモデルではめったに見られない利点です。
On the NanoClimate dataset, the model achieved a MaxSim Accuracy@1 of 0.360, Accuracy@5 of 0.780, and Accuracy@10 of 0.860. Precision and recall scores were consistent, with MaxSim Recall@3 reaching 0.289 and Precision@3 at 0.233. These scores highlight the model’s capability to retrieve accurate results even in longer-context retrieval scenarios.
ナノクライムデータセットでは、モデルは0.360の最大精度@1、精度@0.780、精度@0.860を達成しました。精度とリコールのスコアは一貫しており、Maxsim Recall@3は0.289に達し、精度@3で0.233に達しました。これらのスコアは、長いコンテキスト検索シナリオでも正確な結果を取得するモデルの機能を強調しています。
When evaluated on the BEIR benchmark, GTE-ModernColBERT outperformed previous models, including ColBERT-small. It scored 54.89 on the FiQA2018 dataset, 48.51 on NFCorpus, and 83.59 on the TREC-COVID task. The average performance across these tasks was significantly higher than baseline ColBERT variants. Notably, in the LongEmbed benchmark, the model scored 88.39 in Mean score and 78.82 in LEMB Narrative QA Retrieval, surpassing other leading models such as voyage-multilingual-2 (79.17) and bge-m3 (58.73). These results suggest that the model offers robust generalization and effective handling of long-context documents, outperforming many contemporaries by almost 10 points on long-context tasks. It is also highly adaptable to different retrieval pipelines, supporting both indexing and reranking implementations, making it a versatile solution for scalable semantic search.
Beirベンチマークで評価された場合、GTE-ModernColbertは、Colbert-Smallを含む以前のモデルを上回りました。 FIQA2018データセットで54.89、NFCORPUSで48.51、TREC-Covidタスクで83.59を獲得しました。これらのタスクの平均パフォーマンスは、ベースラインコルベールバリアントよりも大幅に高かった。特に、長いベンチマークでは、モデルは平均スコアで88.39、レンブの物語QA検索で78.82を獲得し、Voyage-Multilingual-2(79.17)やBGE-M3(58.73)などの他の主要なモデルを上回りました。これらの結果は、このモデルが堅牢な一般化と長いコンテキストドキュメントの効果的な取り扱いを提供し、多くの同時代人を長いコンテキストタスクでほぼ10ポイント上回ることを示唆しています。また、さまざまな検索パイプラインに非常に適応し、インデックス作成と再ランキングの実装の両方をサポートし、スケーラブルなセマンティック検索のための多用途のソリューションになります。
Several Key Highlights from the Research on GTE-ModernColBERT-v1 include:This research provides a meaningful contribution to long-document semantic retrieval. By combining the strengths of token-level matching with scalable architecture, GTE-ModernColBERT-v1 addresses several bottlenecks that current models face. It introduces a reliable method for processing and retrieving semantically rich information from extended contexts, significantly improving precision and recall in longer-context retrieval scenarios.
GTE-ModernColbert-V1の研究からのいくつかの重要なハイライトには、次のものが含まれます。この研究は、長時間のセマンティック検索に意味のある貢献を提供します。トークンレベルのマッチングとスケーラブルアーキテクチャの強度を組み合わせることにより、GTE-ModernColbert-V1は、現在のモデルが直面するいくつかのボトルネックに対処します。これは、拡張されたコンテキストから意味的に豊富な情報を処理および取得するための信頼できる方法を導入し、長いコンテキスト検索シナリオで精度とリコールを大幅に改善します。