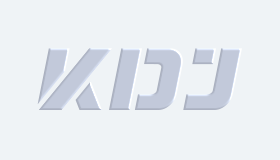
Large language models (LLMs) are revolutionizing natural language processing (NLP) with their ability to generate fluent responses, emulate tone, and follow complex instructions. However, these models still struggle with a critical limitation: they have difficulty retaining information across multiple sessions.
This limitation becomes increasingly pressing as LLMs are integrated into applications that require long-term engagement with users. From personal assistance and health management to tutoring and more specialized tasks, the seamless flow of conversation is paramount. In real-life conversations, people recall preferences, infer behaviors, and construct mental maps over time. A person who mentioned their dietary restrictions last week expects those to be taken into account the next time food is discussed. Similarly, a user who described their hometown yesterday anticipates the LLM to recognize it and use it in later greetings. Without mechanisms to store and retrieve such details across conversations, AI agents fail to offer the consistency and reliability expected from them, ultimately undermining user trust.
The central challenge with today’s LLMs lies in their inability to persist relevant information beyond the boundaries of a conversation’s context window. These models rely on a limited capacity for tokens, which are units of language used by the model, with some models having a capacity of as high as 128K or 200K tokens. However, when long interactions span days or weeks, even these expanded windows become insufficient. More critically, the quality of attention—the model’s ability to focus on and process specific tokens—degrades over more distant tokens, rendering it harder for the model to locate or utilize earlier context effectively. For instance, a user may personally introduce themselves, switch to a completely different topic like astronomy, and only much later return to the original subject to ask for the personally mentioned fact. Without a robust memory system, the AI will likely ignore the previously mentioned details and instead answer based on the last 10 messages, which in this case would be about astronomy, leading to an incorrect reply. This creates friction and inconvenience, especially in scenarios where continuity and accuracy are crucial. The issue is not just about the model forgetting information, but also about it potentially retrieving the wrong information from irrelevant parts of the conversation history due to token overflow and thematic drift.
Several attempts have been made to address this memory gap. Some systems, like those from Google AI and Stanford, rely on retrieval-augmented generation (RAG) techniques. These systems use a separate component to search for and retrieve relevant text chunks from a large knowledge base or prior conversations using similarity searches. Another category of systems employs full-context approaches, where the entire conversation history is simply re-fed into the model at the beginning of each turn. Finally, there are proprietary memory solutions like OpenAI’s Memory API and open-source alternatives like PEGASO, which try to store past exchanges in specialized vector databases or structured formats. However, these methods often lead to inefficiencies. For instance, RAG systems can retrieve excessive irrelevant information, while full-context approaches increase latency and token costs. Proprietary and open-source solutions may struggle to consolidate updates to existing memories in a meaningful way, and they lack effective mechanisms to detect conflicting data or prioritize newer updates. This fragmentation of memories hinders the models’ ability to reason reliably over time.
To address these limitations, a research team from Mem0.ai developed a novel memory-focused system called Mem0. This architecture introduces a more dynamic mechanism to extract, consolidate, and retrieve information from conversations as they unfold. The design of Mem0 enables the system to systematically identify useful facts from ongoing interactions, assess their relevance and uniqueness, and integrate them into a persistent memory store that can be consulted in future sessions. In essence, Mem0 is capable of "listening" to conversations, extracting key facts, and updating a central memory with these facts. The researchers also proposed a graph-enhanced version of the system, denoted as Mem0g, which builds upon the base system by structuring information in relational formats, connecting facts through entities and their properties.
These models were tested using the LOCOMO benchmark, a standard framework for evaluating conversational memory systems. They compared six categories of memory-enabled systems: memory-augmented agents, RAG methods with varying configurations, full-context approaches, and both open-source and proprietary tools. The goal was to assess these systems' ability to process a wide range of question types, from single-hop factual lookups to multi-hop and open-domain queries.
The core of the Mem0 system involves two operational stages. In the first phase, the model processes pairs of messages, typically a user’s question and the assistant’s response, along with summaries of recent conversations. A combination of a global conversation summary over the last hour and the last 10 messages serves as the input for a large language model (LLM) that extracts salient facts. For instance, if the user asks "What is the capital of France?" and the assistant responds with "The capital of France is Paris," the fact extractor would identify "capital_of(France,